Trading Hub
Trading with Precision and Confidence
At Flow Execution, we create tools that transform your trading experience on MetaTrader 5
Execution Modes
Multiple trading modes for any market condition
Risk Management
Set your risk per trade in your account currency
BreakEven
Predefined BreakEven placement by risk-reward ratio
Emotional Control
Trade with confidence and eliminate emotions
Discover more about how Flow Execution can transform your trading with one-click precision, automated risk management, and emotional control features. This isn't just another indicator – it's a complete trading assistant that helps you execute trades with professional-grade precision, reducing slippage and eliminating the emotional decisions that frequently sabotage trading performance.
Today in AI
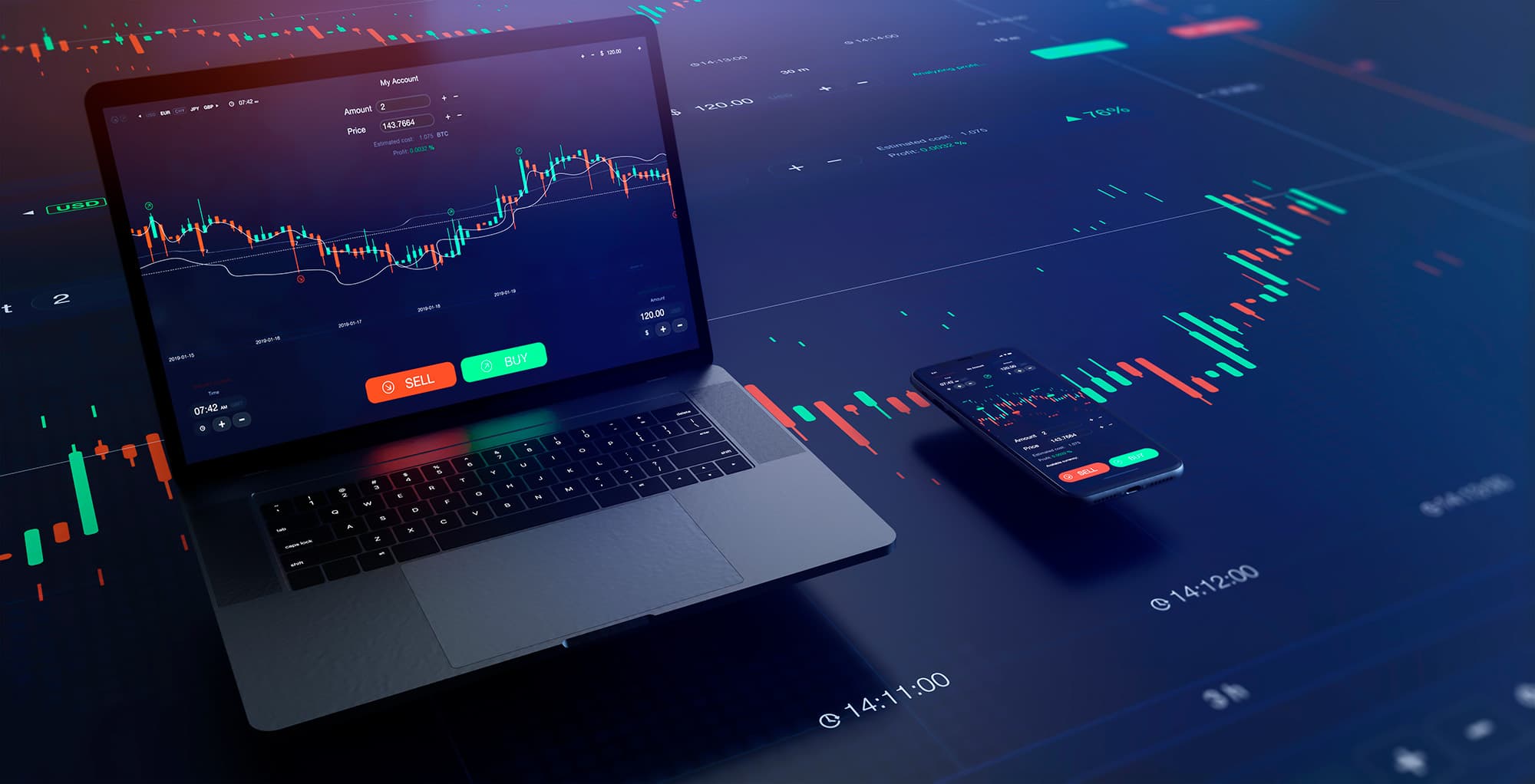
MetaTrader 5: The Ultimate Multi-Asset Trading Platform
Explore articles in this section
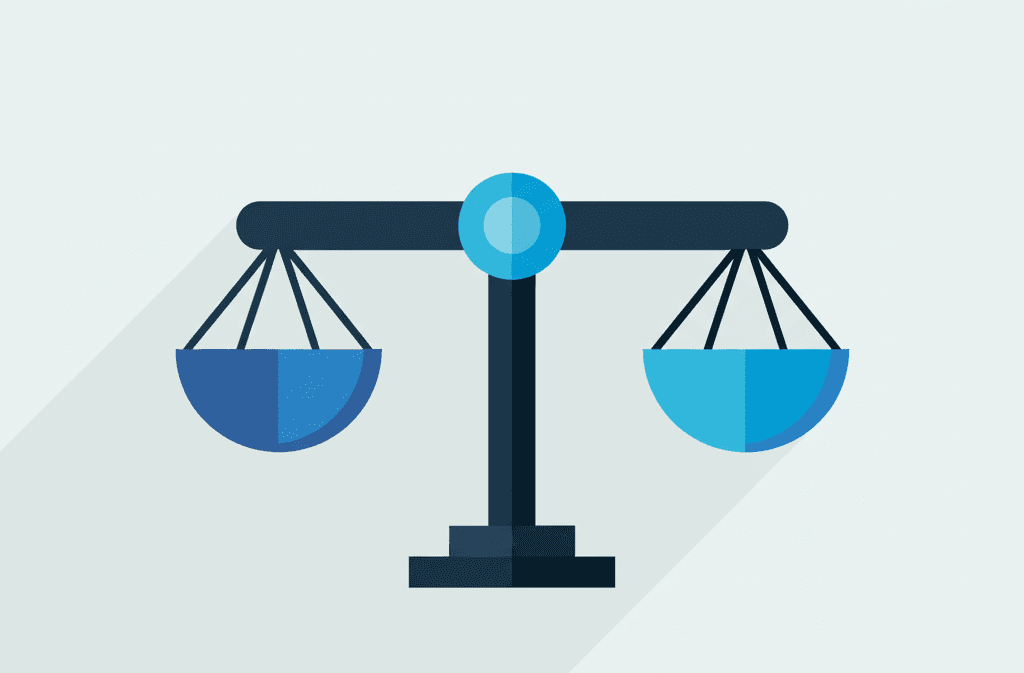
Mastering the Mental Game: The Trading Psychology Behind Success
Explore articles in this section
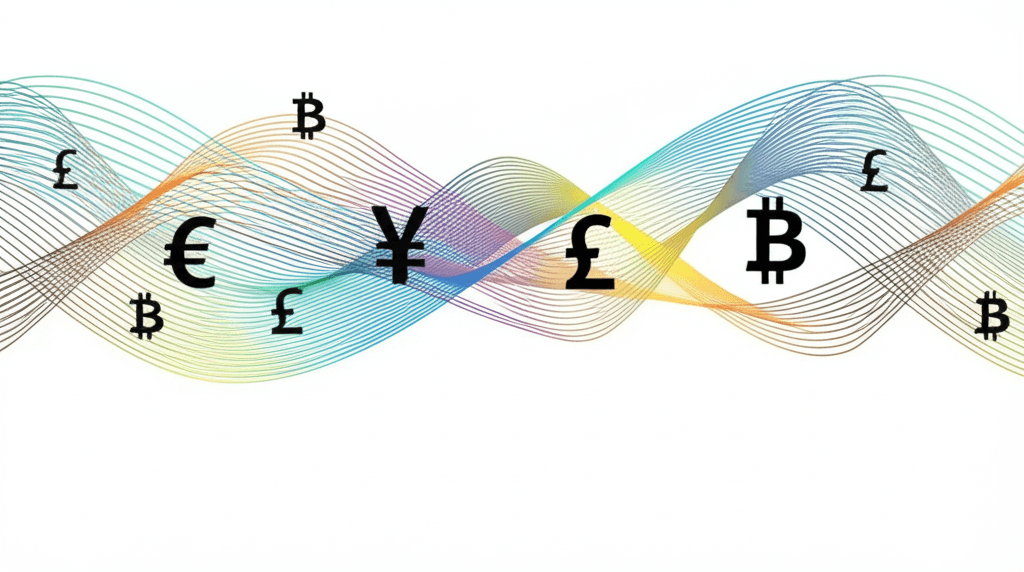
Forex Trading: Mastering the Global Currency Market
Explore articles in this section
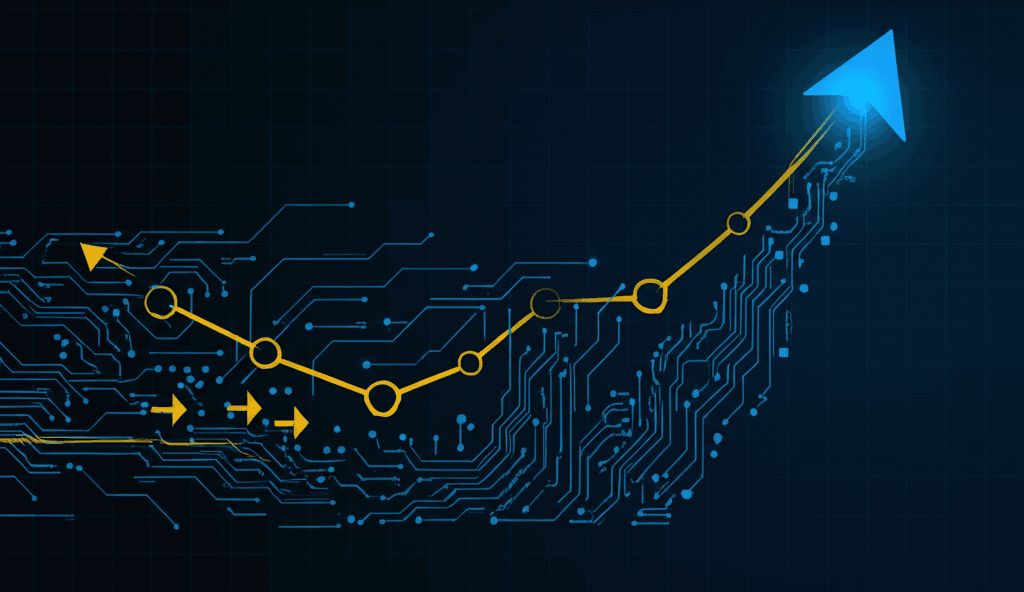
The Evolution of Autotrading: AI, Algorithms, and Market Access
Explore articles in this section
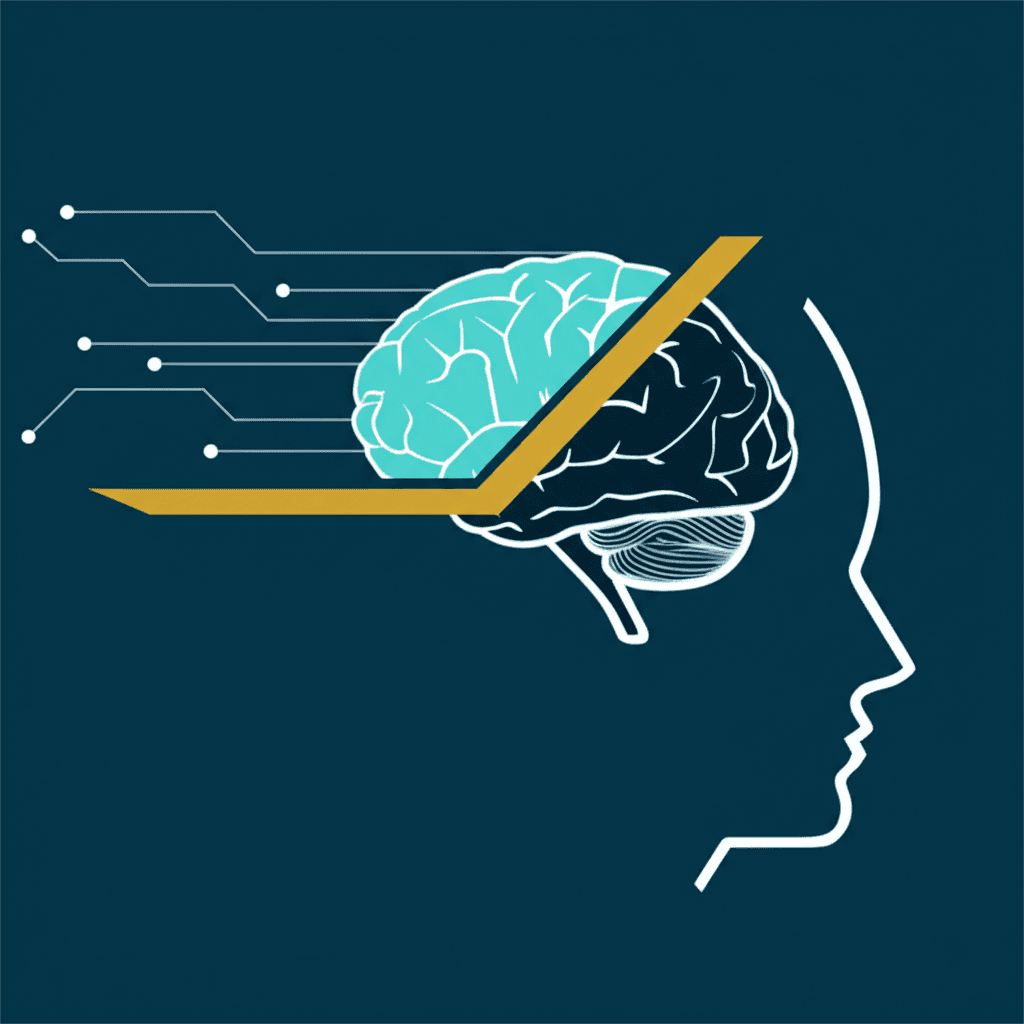
Day Trading Mastery: Strategies, Platforms, and Market Insights
Explore articles in this section
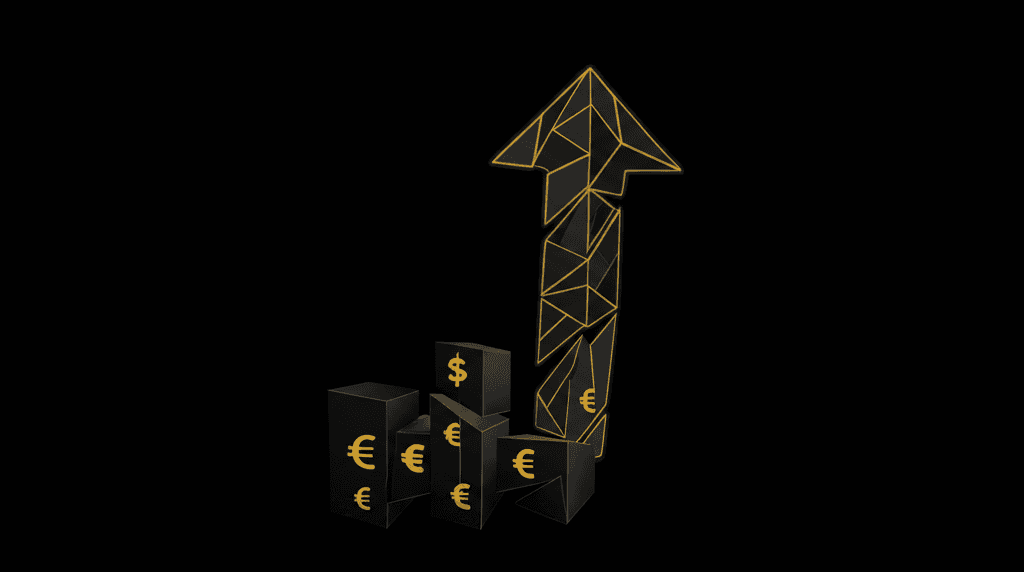
FTMO: The Evolution of Proprietary Trading in Modern Markets
Explore articles in this section
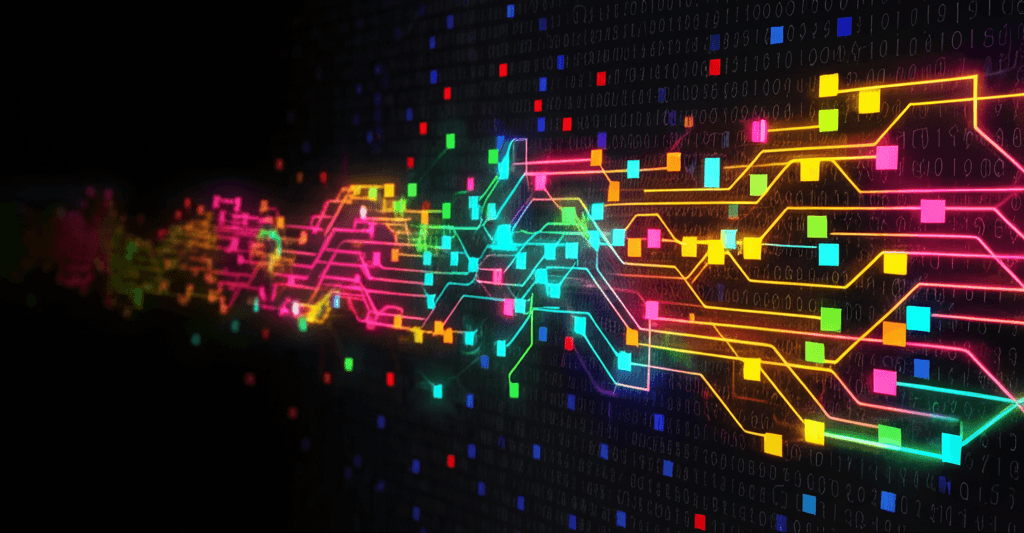
Expert Advisors: Automating Success in Financial Markets
Explore articles in this section
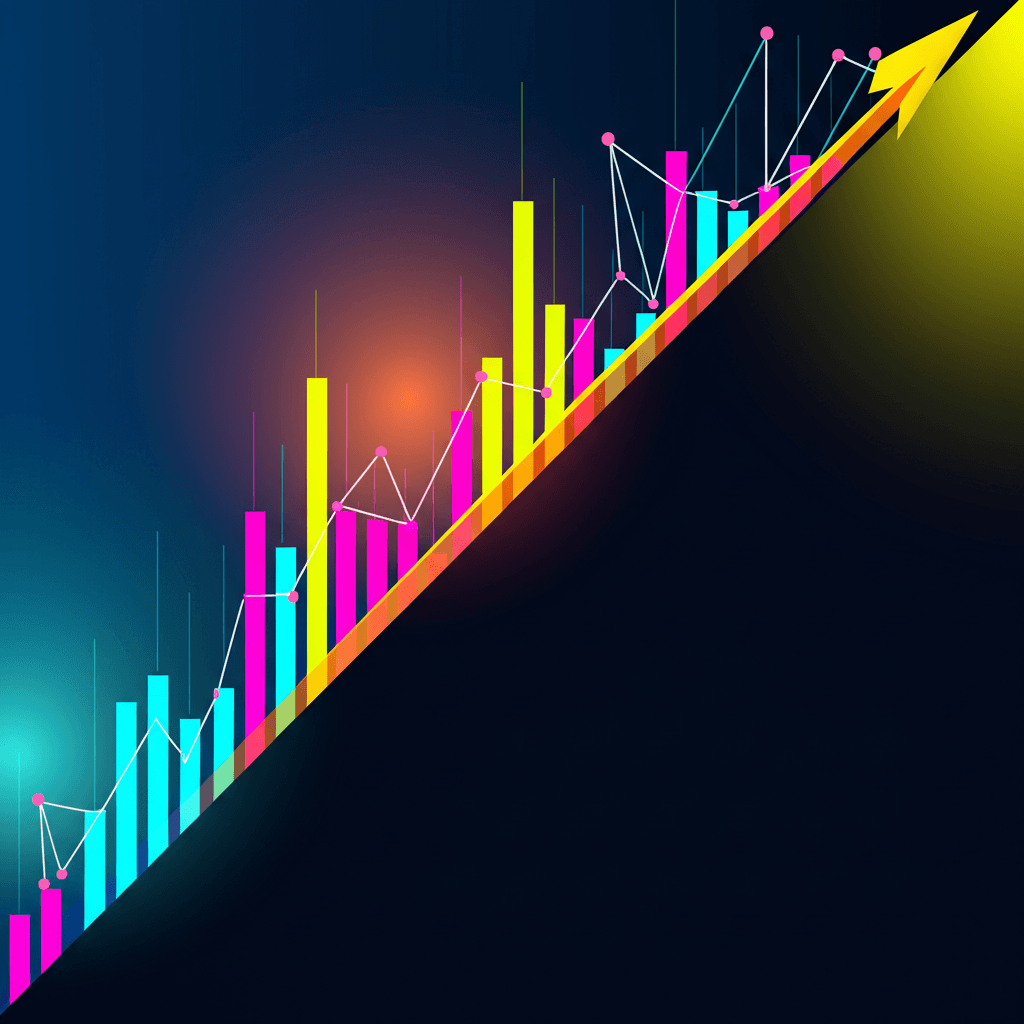
MetaTrader 4: The Trading Platform for Forex Investors
Explore articles in this section
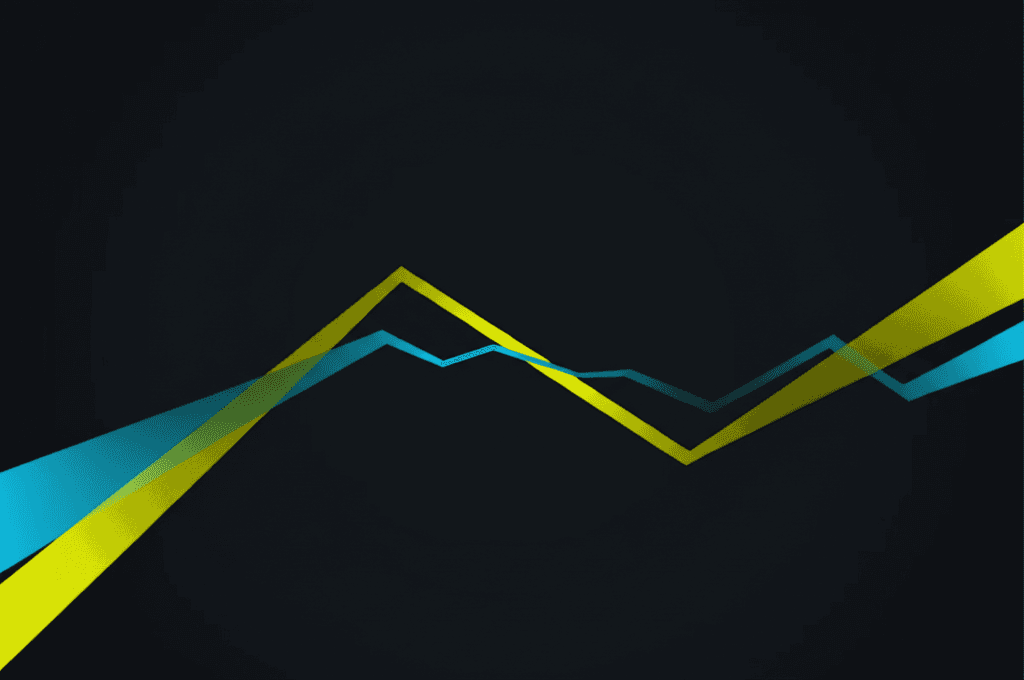
Oanda: The Complete Foreign Exchange and Trading Platform
Explore articles in this section
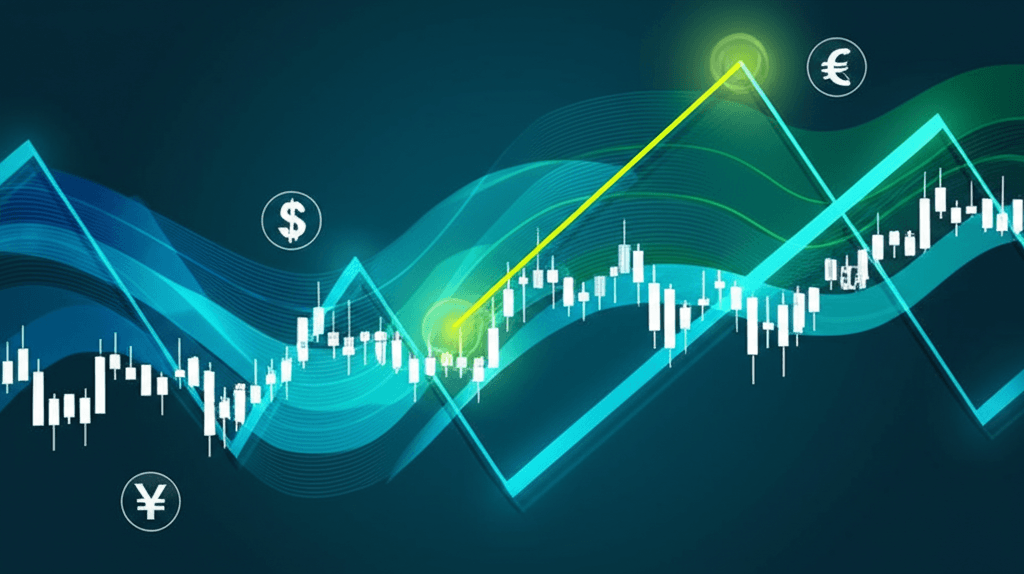
Forex Signals: Mastering Currency Trading with Expert Alerts
Explore articles in this section
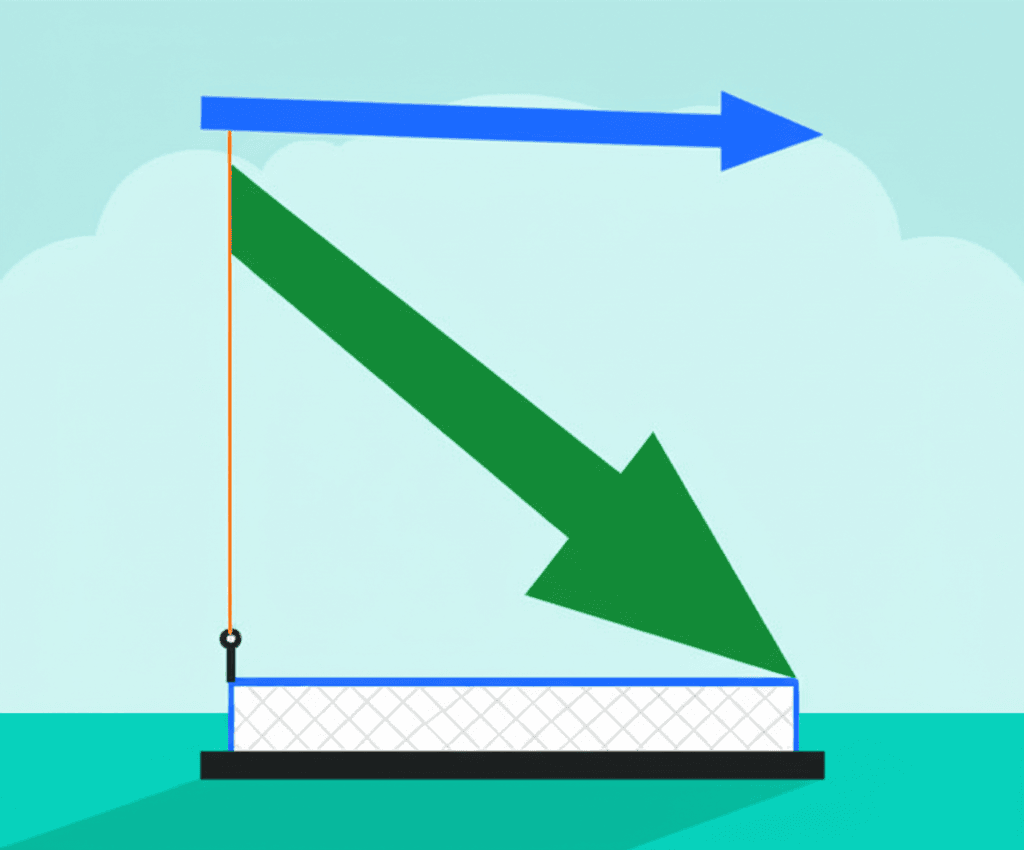
Stop Loss : Essential Trading Risk Management Strategies
Explore articles in this section
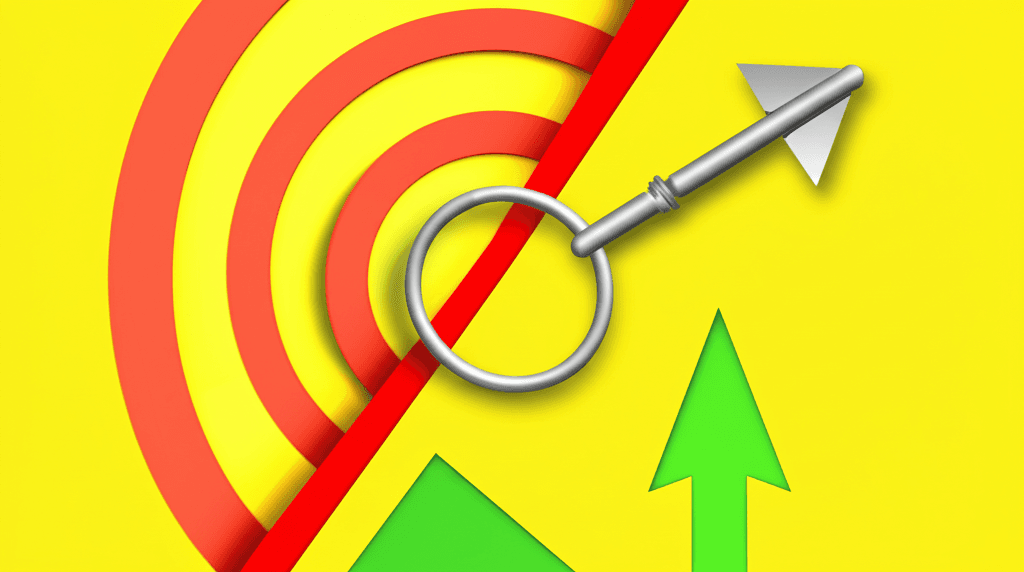
Take Profit: Advanced Strategies for Profit Maximization
Explore articles in this section
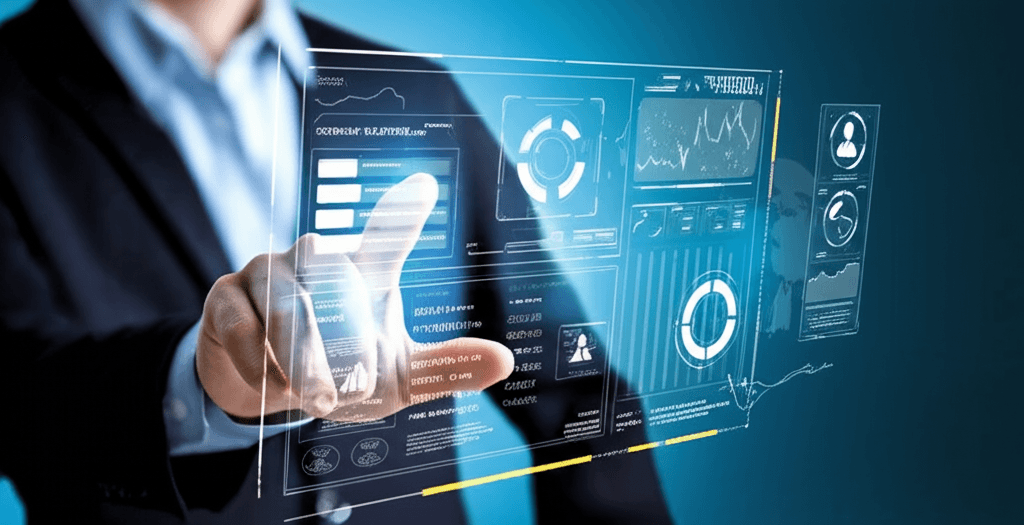
Trade Assistant MT5: Advanced Trading Tools for MetaTrader
Explore articles in this section